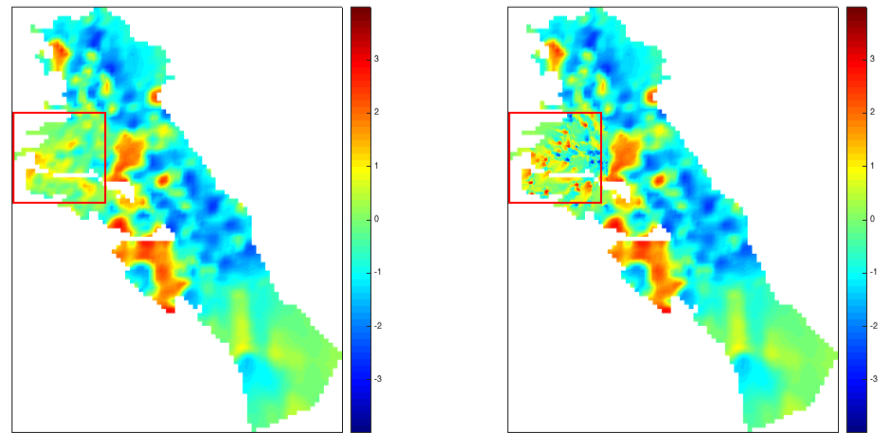
Artificial neural network for large-scale liquefaction prediction
Artificial neural networks (ANNs) are widely used in spatial analysis and predictions of geotechnical and other engineering problems. For example, ANN is used to evaluate the spatial variability of rock depth in an extended region [1]. Prasomphan and Mase [2] develop a scheme to generate prediction map for geostatistical data using ANN. When integrated with GIS geographic information system (GIS), ANN is a very powerful tool to make spatial analysis. For example, Ryu et.al [3] integrated with ANN to predict the regional landslide susceptibility.
It is surprising that ANNs are rarely found to be applied to regional liquefaction assessment while they have been used extensively in spatial analysis. Therefore a research topic is proposed to investigate into the combination of ANN with multiscale random field for regional liquefaction prediction. The benefit will be improvement of prediction accuracy with combination of the advantages of both methods. The challenge is to develop an ANN that is aware of multiscale spatial variability.
I trained a single scale ANN for liquefaction prediction in East Bay, San Francisco. It works well but still need improvements. Work is going on to make the ANN work with multiscale random field.
[1] TG Sitharam, Pijush Samui, and P Anbazhagan. Spatial variability of rock depth in bangalore us- ing geostatistical, neural network and support vector machine models. Geotechnical and Geological Engineering, 26(5):503–517, 2008.
[2] Sathit Prasomphan and Shigeru Mase. Generating prediction map for geostatistical data based on an adaptive neural network using only nearest neighbors. International Journal of Machine Learning and Computing, 3(1):98, 2013.
[3] Saro Lee, Joo-Hyung Ryu, Kyungduck Min, and Joong-Sun Won. Landslide susceptibility analysis using gis and artificial neural network. Earth Surface Processes and Landforms, 28(12):1361–1376, 2003.
···